Digital America interviewed Dennis Delgado in April 2024 about his series The Dark Database (2019-2020).
:::
Digital America: In your series, the Dark Database, you confront white supremacy and racial bias embedded in biometric facial recognition. How were you introduced to facial recognition? What inspired you to create art on the subject?
Dennis Delgado: I was introduced to facial recognition primarily through Simone Browne’s book Dark Matters: On the Surveillance of Blackness, although I had been tangentially interested in surveillance, race and practices of looking in general for a long time. One of the things that is difficult to deal with on a daily basis is being “seen” as a person of color. I should say I am Puerto Rican. My mother was raised in Puerto Rico and my father grew up in Spanish Harlem. It is chilling when people do not recognize you after you have had multiple interactions with them sometimes. I have had people hire me to teach and a week later confuse me with an undergrad student or another person of color. I’m sure it is benign in some instances; however, in the back of your mind you cannot help but wonder if people are just demonstrating their internalized sub-conscious biases. The entire culture in the United States is decidedly Eurocentric and anti-Black (amongst other things) and so it is easy for anyone, even people of color, to internalize white supremacy. Furthermore, I found it fascinating that now that artificial intelligence is being incorporated into surveillance systems that we are not only teaching computers to “see,” but teaching them a particular way to see. I had been making a series of videos on the ethnographic display of Ota Benga at the Bronx Zoo (in 1906) and was struck by the use of the zoo cage as a “technology of vision.” Ota Benga an (African Mbute tribesman) was presented as an intermediary between man’s primate ancestors and the modern European white male. This was done in the context of Darwin’s then popular Origin of the Species. I recognized a corollary between the discursive othering of Africans and the present-day “othering” of facial recognition. It was then that I felt like I had to try to make something that addressed the issue.
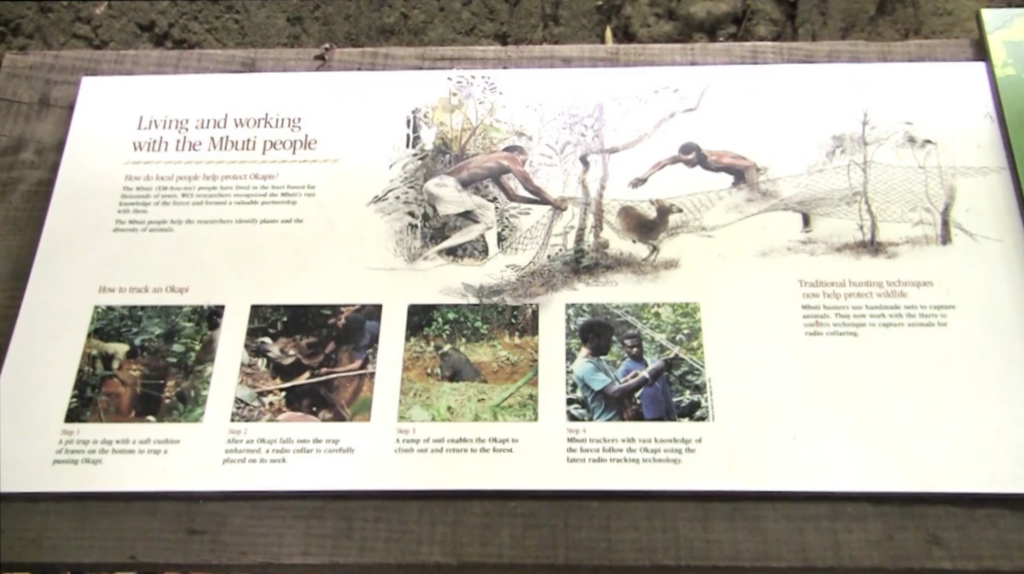
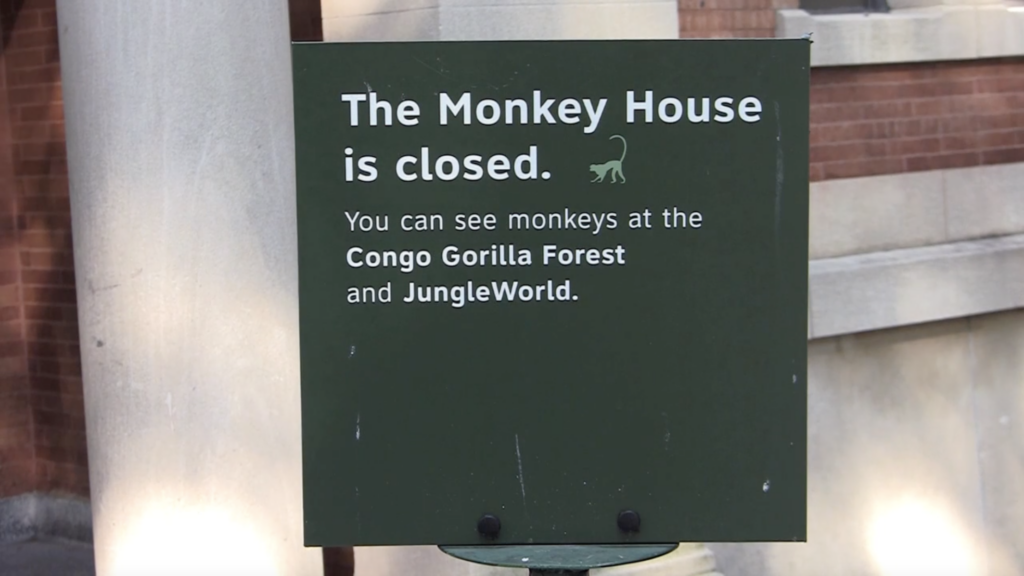
DigA: The Dark Database reveals the lack of visibility of people of color within algorithms—AI’s failure to read faces with darker complexions. The portraits create an image so overwhelming with identity that the generated face is unreadable; however, to the human eye, the showcasing of Caucasian features evidently outweighs invisibility. Examining the issues of the technology’s inability to acknowledge skin tone or race, what were your initial predictions for this research? What are your hopes for the future in terms of the technological development of artificial intelligence functions?
DD: There are dozens of studies on facial recognition systems that reveal that those systems have not been trained properly, i.e. the datasets which were used to teach those systems were not diverse enough to encompass a wider range of skin tones. In others words the Open Computer Vision facial detection algorithm was suffering from its own sheltered space of development. The systems had not had any exposure to equivalent numbers of faces from people of color. Initially I thought the OpenCV algorithm would function much more predictably than it did and I tried to include its mistakes in the final work as well as its accurate hits. There were instances where the algorithm detected a face in the landscape or confused an animal with a human face (specifically a taxidermized deer in the film Get Out). In another film the algorithm detected faces in the purely graphical black-and-white title sequence of John Singelton’s Higher Learning. I was genuinely surprised at how the algorithm performed. One of the things pointed out in Simone Browne’s book, Dark Matters, was that these systems were “probabilistic” in nature and rely on comparison, and therefore never one hundred percent accurate. Facial recognition relies on comparisons of vectors. If you want it to identify me “accurately,” you have to feed the system as many images of myself as available. As the system is trained, the idea is that the vector representation generated by it to map my face will be adjusted as it learns more about what I look like from additional images. My hope for the future is that the use of facial recognition be federally regulated, and that that regulation set a minimum number of dataset images that must be used and tested before it is deployed or sold to law enforcement or any interested party. There is a lot of pressure to monetize and distribute the technology, and things like our iPhones and Adobe programs are already employing these algorithms. States like California understand the risks, and have taken actions to regulate their use. I hope that continues on a national and perhaps global level. Because the generation of “real-value feature vectors,” uses advanced mathematics, these platforms have an appearance of objectivity, but they are not objective and should not be used until further development takes place.
DigA: While detecting people of color, facial recognition technologies are more prone to making errors in the process of confirming identification. This is seen as an issue in law enforcement; flaws in facial recognition perpetuate inequities in policing, resulting in wrongful cases, arrests, and convictions. To escape visibility, Zach Blas’ Facial Weaponization Suite similarly challenges surveillance by merging facial recognition data to construct an undetectable face mask. Projects like this can begin to illuminate a new way of thinking: could a lack of facial recognition be a good thing?
DD: I think yes, like Ralph Ellison stated in Invisible Man, there are advantages to not being “seen”; however, it is worth stating that in the case of recognition or misrecognition it only adds to the suspicion and categorization that people of color already face on a daily basis, particularly in the realms of law enforcement. The abstraction of the system and its concealment of bias under a veneer of objectivity and mathematics makes it a particularly dangerous political tool. Already there have been at least a hand full of reported instances of facial recognition misidentifying suspects who are African-American. Luckily those incidents did not escalate or result in injury or death, but the potential for that to happen is frighteningly real. I am not completely sure that a lack of facial recognition is a good or a “bad” thing. I think it is a tool; however, that is in need of revision and regulation. It is a powerful tool, and it may prove useful, but for the time being it remains problematic.
DigA: Looking at your series Mbye, composed of photographs, archival materials, and short films that document the crimes of dehumanization, you shed light on Ota Benga’s disturbing story of zoo captivity by tackling themes of colonialism and surveillance in the United States. How do you see these themes develop into your current work? What parallels did you find when comparing monitoring systems of zoo experiences with more recent AI technologies?
DD: My project on Ota Benga remains close to my heart in a strange way. I was deeply saddened by learning about his story and the history of ethnographic display in the United States and specifically in New York City. I felt compelled to try and make sense and come to terms with that history by trying to making the artworks about it. At around the same time, Black Lives Matter began to took form, and a new visibility of deadly force being used against African-Americans also re-entered the national consciousness. I started to see the lines of connection between the treatment of Ota Benga and people of color today. In the case of Ota Benga, the technology used to shape New Yorker’s perceptions of African people as inferior and less than human, was the zoo cage. The cage provided a division and simultaneously a visibility which set the stage for a particular practice of looking. To quote Lisa Cartwright and Marita Sturken,”the gaze is [conceptually] integral to systems of power, and [to] ideas about knowledge.” Ota Benga was presented as a link between our primate ancestors, and the modern Eurocentric homo sapien. Instead of being seen as a sophisticated and intelligent man removed and misrepresented within another culture. The same practices exist today. The Wildlife Conservation Society still maintains a relationship with the Congo. It still appears to impose its own agenda on Mbute Tribes people prioritizing the preservation of gorillas in the Congo, over those of its local Mbute inhabitants. Mbute tribesman’s knowledge and culture is presented alongside gorillas, creating a kind of equivalency that is still distorting knowledge for viewers today (over a hundred years later). I found the same corollary in facial recognition systems. Developers of Open Computer Vision (since the 1960s) suffered from the same Eurocentric myopia that zoologists indulged in in the late 19th and early 20th centuries. Within facial recognition systems people of color are discursively digitized, reconstructed, and conceived as “other”, as well as categorized as less recognizable and therefore suspect. The same treatment, or actually worse was afforded to Ota Benga. As Kelly Anne Gates points out in her book Our Biometric Future: Facial Recognition Technology and the Culture of Surveillance, facial recognition systems are cultural products that reflect particular “ways of seeing” some of which go back centuries in the making.
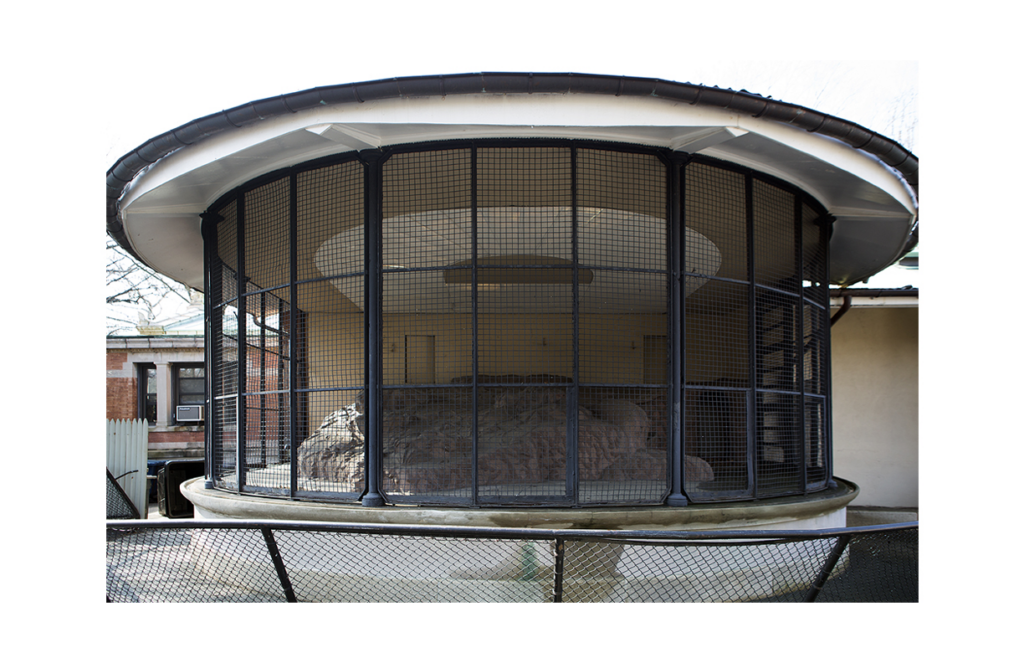
DigA: Your work tends to break the boundaries of artificial intelligence art’s controversies. What techniques and tools are used to form these images, and could you describe your creation process of the Dark Database? What are your thoughts on artificial intelligence generated art?
DD: For the Dark Database series, I used Open Computer Vision. OpenCV is a series of open-source libraries used with the programming language Python to process images, detect objects, faces, motion, etc. OpenCV contained facial detection and facial recognition components, and I learned that I could install and run these scripts to detect, categorize, and label faces. It was a somewhat steep learning curve (as I am not a coder), but I did feel comfortable as a computer technician installing and trying out the software. After a long learning process of trial and error, I was able to run facial detection scripts and learn more about Python. I then started reaching out to students (at Cooper Union at the time) to try and get help customizing scripts to process video. I had the script export a cropped .jpeg image of each face it detected in every 30 frames (or every second) of a film. I deliberately chose films by directors of color where the lead male role in the film was played by an African American actor (and later Hispanic actors). I also tried to limit myself to films that were about policing people of color or about black masculinity. I wanted to see how the algorithm would perform in these contexts. Each film yielded anywhere from 300 faces to 700 faces each with a particular range of skin tones. I then stacked those faces in Adobe Photoshop, centering and resizing them to align with a simple and centered portrait-template. I then used Photoshop to calculate the median pixel value for the skin tone of each detected face. The result was this at times blurry abstraction of a face. The image struck me and resonated on a number of levels. It appeared ghostly, and in my research, I encountered Derrida’s concept of Hauntology which I felt the image visualized in a dramatic way. I felt like I was, to a certain extent, uncovering the ghost within facial recognition systems which spoke to the re-emergence of suppressed racism in our visual culture. There were also direct relationships between Francis Galton’s photographic facial composites from the late 19th century. The use of photographic technologies to legitimize the eugenic and taxonomic practices of phrenology and physiognomy. I was pretty thrilled with the results, and could not wait to try the technology on other films. The project also reminded me of the ease with which everyone in our culture (including people of color) can internalize white supremacy often unconsciously. I am continuing to look at Open Computer Vision and looking at how the systems are trained to see, as well as the importance of diversifying datasets to improve on current systems. As far as artificial intelligence, I do not see it as a threat to artists. I see it more as a cultural product in and of itself. It reflects many of the biases and ways of thinking and visualizing that stubbornly remain in the culture. Further, I think I still side with Picasso in that computers alone are “useless,” as they provide only answers but I am still interested in the questions computers can help artists pose. AI is modeled on the neural structures of our brain; however, I think the specific region of the brain being modeled is more important. In a decidedly right-handed, left-brained, and logical world, I think it is important to recognize that human beings, and artists specifically, still occupy and employ the right sides of their brain and that is the thing we still have to offer. AI synthesizes images from the vast troves of image datasets and algorithmic assumptions that have been already compiled, created, imagined and visualized, but what about what we have not imagined or visualized yet? I am speaking in generalized terms, but I think artist’s own organic algorithms are more interesting to me. What Van Gogh saw in the night sky, the emotion he infused into a self-generated image with his innate understanding and observation of color and his synthesis of all of this information is what we still look at in wonder.
DigA: Can you talk about any upcoming projects you are working on now?
DD: Lately I have been looking at Monocular Depth Estimation (a specific area in computer vision) and trying to see if our culture’s obsession with African-American athletes (specifically NBA players) can be visualized or understood through the optical and conceptual lens of computer vision. I am not sure if this will lead to anything, but I have been looking at Allen Iverson (a now retired basketball player) as an interesting contemporary example of what I understand as “Unforgiveable Blackness.” To use a term taken from Ken Burn’s documentary on Jack Johnson. Allen Iverson’s refusal to integrate into the corporate culture of the NBA made him both a target of the mostly Caucasian-run National Basketball Association and simultaneously a hero amongst a younger generation of both fans and players steeped in the culture of Hip-Hop. I have been running Monocular Depth Estimation scripts on video highlights of Allen Iverson’s basketball career and am interested in how the images are processed for Depth and motion detection, and I am trying to create a video that sheds some light as to how things like automated cars, etc. will view people of color. Are they present and “visible,” with in the context of the urban landscape? Are we teaching robots and automated cars to see the “other” differently? If we slow down video clips of professional athletes, does it affect the ways these technologies see? Is there a ghost waiting to present itself within the spectral space of Monocular Depth Estimation? These are amongst the most recent questions I am trying to explore.
:::
Check out The Dark Database (2019-2020) by Dennis Delgado
:::
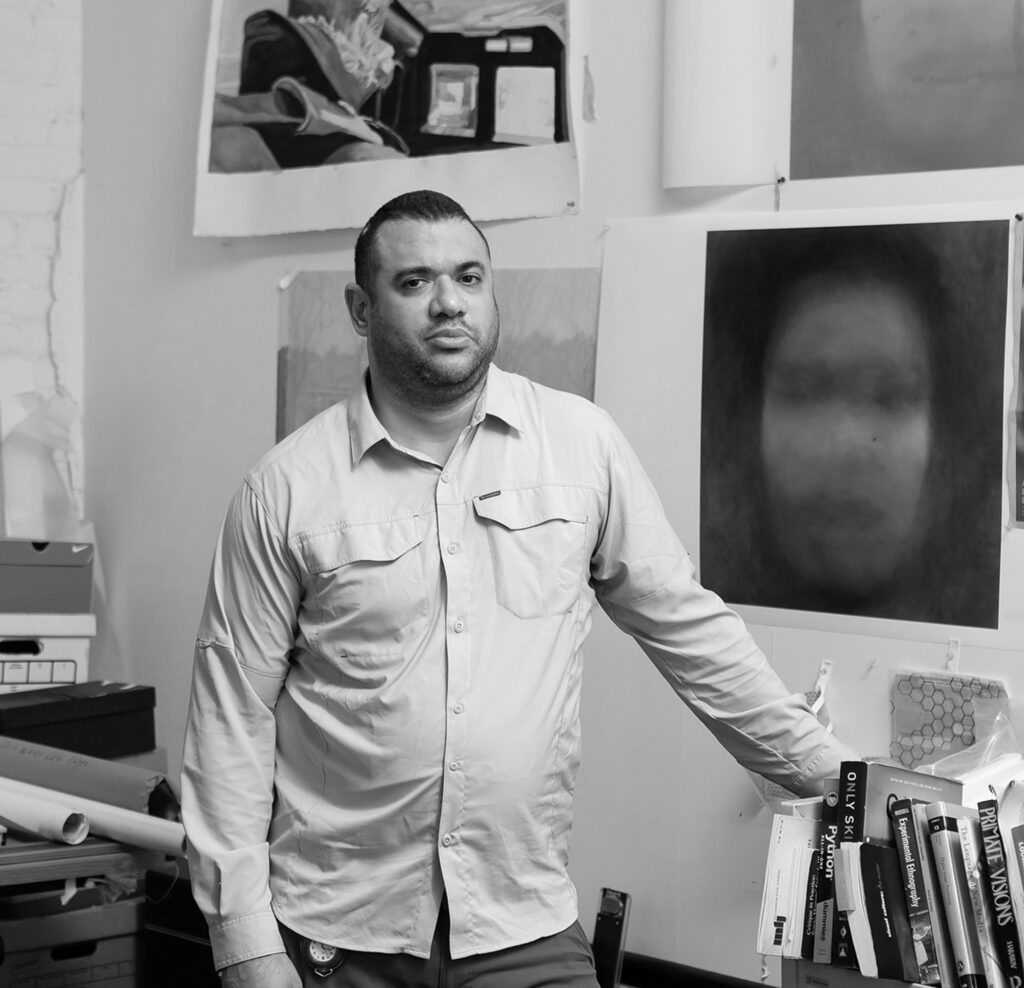
Dennis Delgado is a Bronx-born multimedia artist interested in technologies of vision, analysis of persistent colonialist ideologies, and regimes of expansionism. He has received a BA in film studies from the University of Rochester, as well as an MFA in sculpture from the City College of New York. His work has been exhibited at the Bronx Museum of the Arts, UC Irvine, and the other.